Calendar Variables in
Marketing Mix Modeling
Calendar Variables in
Marketing Mix Modeling
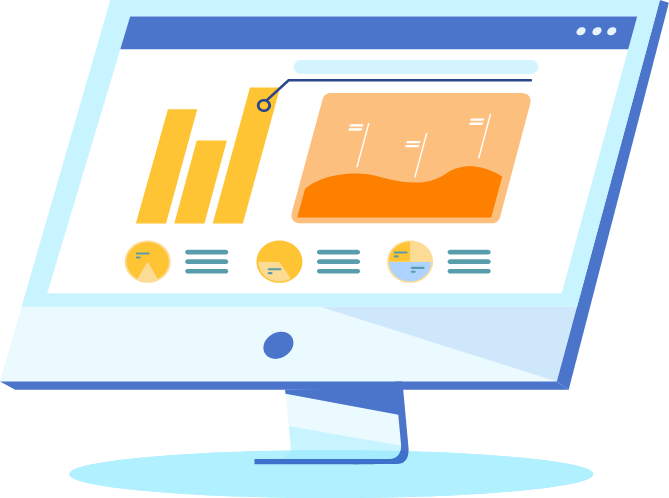
A successful marketing mix modeling project necessitates a profound grasp of statistical and business knowledge.
A crucial aspect of this endeavor lies in choosing the right variables that accurately reflect consumer behavior and market reality, including the incorporation of calendar variables.
In this article, we delve into the significance of calendar variables in marketing mix modeling, highlighting how their integration can boost model accuracy, statistical robustness, and real-life relevance.
By leveraging MMM insights, businesses can gain a deeper understanding of their customers, make well-informed marketing investments, and maximize their return on investment (ROI).
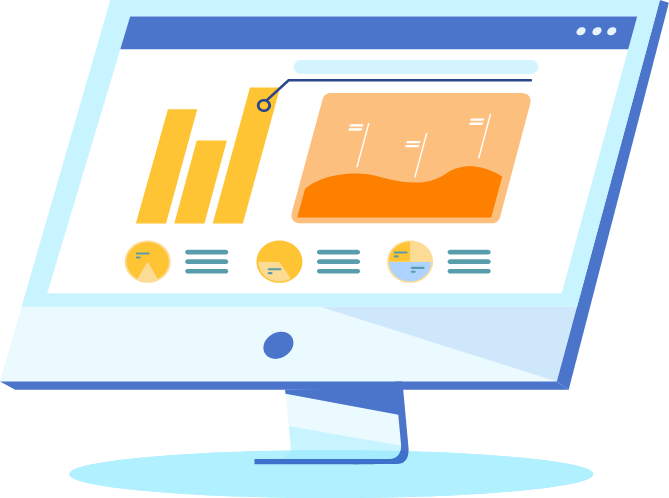
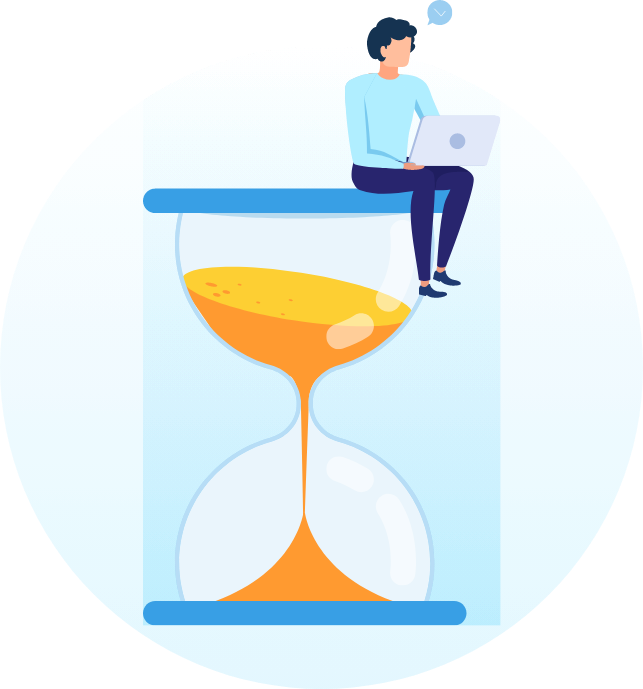
Understanding Calendar Variables:
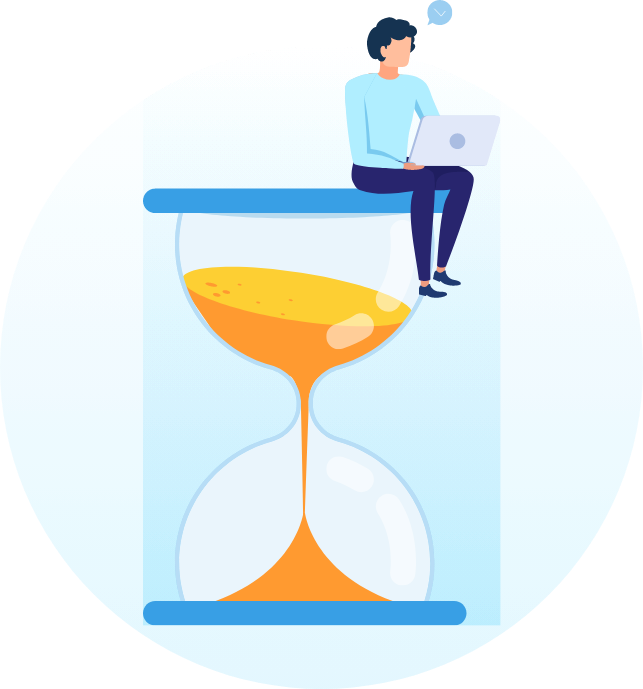
Calendar variables are generally time-related factors that impact consumer behavior and purchase patterns. They are used to model the influence of external and uncontrollable factors (Product out of stock, Special discount, Network problems, etc.) or specific events (Christmas, Black Friday, Payday, etc.). By incorporating calendar variables into marketing mix models, businesses can gain valuable insights into how different time periods affect their marketing outcomes.
The first type of calendar variables is dummy variables.
Let’s Get Specific about Calendar Variables
The first type is dummy variables.
A dummy variable is a binary variable that represents/models the occurrence of an event in a particular period (e.g., week). For instance, if a product went out of stock for one period (see figure 1), a dummy variable can be created to capture the impact of the stock shortage during that specific period.
In this article, we explore the significance of calendar variables in Marketing Mix Modeling, highlighting how their integration can boost model accuracy, statistical robustness, and real-life relevance.
By leveraging MMM insights, and the right MMM software, businesses can gain a deeper understanding of their customers, make well-informed marketing investments, and maximize their return on investment (ROI).
Figure 1: Dummy variables showing the day the store went out of stock
Careful though! Dummy variables should be used cautiously. Creating too many dummy variables without understanding their significance can lead to overfitting the model.
Window Variables:
Window variables are created to model factors or variables that span over time.
Unlike dummy variables, which represent a single period, a window variable encompasses a sequence of dummies to account for events that occur over an extended period. For example, if a retailer store is closed for refurbishing for a period of time, a window variable can be created to capture the impact of the store refurbishing.
Figure 2: Window variable representing Store Closing Period
Another example is modeling the effect of covid, where a window variable can be used to represent the covid period and model its impact on sales.
Interval Variables:
Interval variables are a concatenation of windows.
For example, it could be employed to represent different promotional periods or events that occur at specific times. For instance, if a retailer runs four promotional periods during the modeled period (see figure 3), an interval variable with four windows can be created to capture the effect of promotions. This allows for disentangling the promotional impact on sales or revenue.
Figure 3: Interval variable showing the impact of Fall and Spring Promotions
Trend Variables:
Trend variables are used to model the gradual increase or decrease of a specific Key Performance Indicator (KPI).
For example, if market growth is impacting both own and competitors’ sales a trend variable can be created to model this effect.
It’s crucial to have supporting variables that justify the creation of the trend.
Figure 4: Trend Variables
Trend variables are used to model the gradual increase or decrease of a specific Key Performance Indicator (KPI).
For example, if market growth is impacting both own and competitors’ sales a trend variable can be created to model this effect.
It’s crucial to have supporting variables that justify the creation of the trend.
Periodic Variables:
Periodic variables are used to model repetitive events, such as paydays, monthly seasonality, etc.
By deploying periodic processors, a variable can be created to indicate when a specific day falls within a week. For example, a variable can be created to represent the 25th of each month, which would be active (take a value of 1) when that date “25th” occurs in a particular week (see figure 4). This variable can then be used to analyze the impact of payday on sales (assuming that people tend to spend more when they receive their salaries).
Figure 5: PayDay Calendar Variable
Conclusion
Introducing calendar variables into a marketing mix model enables businesses to gain deeper insights into the impact of time-related factors on their marketing variables. Whether through dummy variables, trend variables, window variables, interval variables, or periodic variables, understanding and utilizing calendar variables can enhance the accuracy and effectiveness of marketing strategies.
MassTer, MASS Analytics’ Marketing Mix Modeling Software, was built with calendar variables in mind. The software contains a dedicated module for data processing. It includes easy-to-use features to create different types of calendar variables. By considering the nuances of different time periods and events, businesses can optimize their marketing mix and achieve better results.