Get an Optimized Marketing budget using Geo-based A/B testing in 6 Steps
As a marketer, you operate within a predefined annual budget for managing diverse marketing channels and launching campaigns. This often offers minimal room for errors, especially when dealing with substantial budgets.
Enter A/B Testing, a pivotal tool that enables you to strategically allocate your available budget, seeking the optimal investment distribution among regions.
In this article, our focus lies on Geo-based A/B Testing, where we provide a comprehensive guide on:
- Crafting your Geo-based A/B Test.
- Quantifying results through Pooled Regression in Marketing Mix Modeling.
We will lead you through the essential six steps to master this process effectively.
Step 1: Define your Hypotheses
In this first step, you would need to decide what test you would like to run in relation to your media execution. Depending on your context, this can either be testing a new media channel, using a new copy format (6s video vs. 10s video), or running a new campaign. The context we will be addressing is that of a Skincare company wanting to invest in Digital Out of Home (DOOH) as a new media channel. The hypothesis here is that investing in DOOH will bring additional revenue.
To test this hypothesis, a feasibility study needs to be done. And that’s your next step!
Step 2: Feasibility Study, Principles
When designing your Geo-based A/B Test, it’s important to note that the below 4 factors are key to obtaining quality results:
- Sales Impact:
The greater the uplift in transactions/sales caused by an activity, the easier it is to measure. In our example (see figure 1), the commercial return generated from running DOOH Ads should be significant. Keep in mind that the revenue generated is only accounted for during the testing period so it’s considered a short-term revenue.
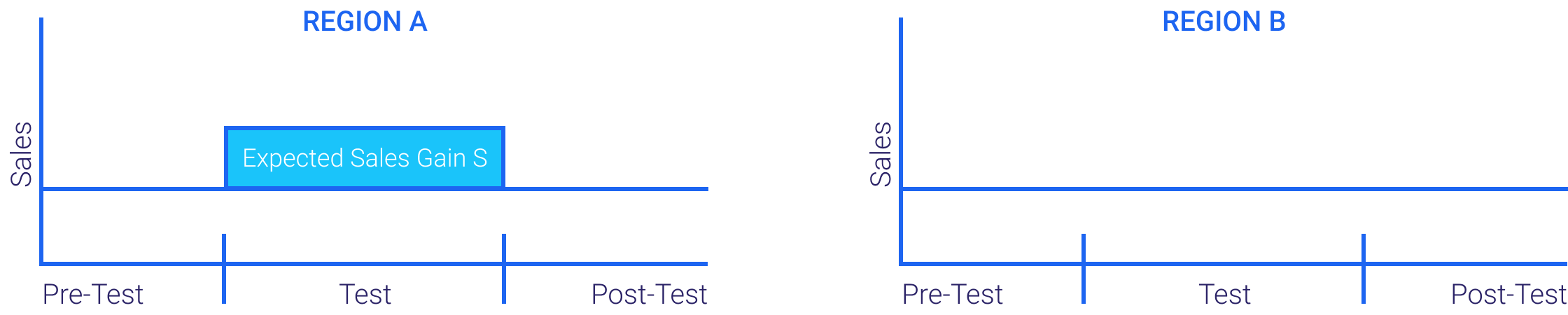
Figure 1: Sales Data from Region A and B during the A/B Test
2. Testing Factor Variations
The greater the variation in the testing factor (e.g., advertising, BTL, etc.), the easier it is to measure. To ensure that the variation is unique to the testing factor, it’s important that regions selected for test and control are similar demographically (population, strength of brand, etc.)
3. Period
The greater the number of periods of data, the easier it is to measure. To ensure this, it’s important at the beginning of designing the Geo-based test to determine:
a. How long would you like to run the test?
b. In which regions would you like to run the A/B test?
4. Sales Variations
The greater the variation in the transactions/sales data, the more difficult it is to measure.
These 4 factors will directly influence the level of confidence “C” of your measure. Choose your level of confidence wisely as it will be the metric that will help you balance being reasonably confident and minimizing the risk of false negatives.
Step 3: Design your test: Select test and control regions
Now that your hypothesis is clear and that you have decided what confidence level you would like to opt for, it’s time to get to work!
As mentioned previously, the regions you select for your test should be fairly similar to minimize the impact of uncontrollable variables in the test. In the context of our Skincare company, we would like to run DOOH in the Midwest region of the US. Knowing that the states of Illinois, Ohio, Michigan, and Wisconsin have some demographic similarities, they are selected as test and control regions.
- Michigan and Illinois are the “test” group where we will be implementing the DOOH Ad.
- Ohio and Wisconsin are the “control” group where we don’t implement any changes.
Step 4: Run your Test and Collect Data!
This is one of the most crucial steps in the process because if you don’t have data, you can’t track and measure. Make sure that data is available for both test and control regions over a defined period. Data for the before, during, and after the A/B test period will provide the best insights about the effectiveness of your media investment in the hypothesis.
Step 5: It’s time to run your Marketing Mix Modeling Analysis!
Based on the data you have gathered; you will be building Marketing Mix Models to assess the effectiveness of your A/B Test. Using the pooled regression technique, you will be using data for your test and control regions to analyze results. In our example here, you would build a pooled sales model for the skincare product in the Midwest and break your results down by region.

Running Pooled Regression for 4 regions can be a challenge; it’s important to select the right tool that will enable you to recognize the variations across the different regions and get insights into the effectiveness of implementing DOOH Ads in the test regions compared to the control regions.
Step 6: Draw conclusions
The results derived from your Marketing Mix Modeling analysis will enable you to decide whether to reject or deny the hypothesis we defined in Step 1. But this doesn’t mean to jump straight into action!
If the results of the A/B Test we launched initially were positive and DOOH turned out to be a revenue-generating media, we could consider implementing DOOH Ads in the control regions. It’s important to keep in mind that as a marketer with a knack for statistics, you should always be alert for any changes that could occur between the regions and the periods where the test was designed and run to get the best results.
In case the results are negative, and the hypothesis was rejected, refining the hypothesis, and repeating the A/B testing process are an option intended to further optimize marketing media strategies.
To sum up, running a Geo-based A/B test is a process that requires much planning and that can be challenging to measure. When embarking on this journey, your best friends are:
- A critical mind and a good understanding of the market you’re operating in.
- A strong statistical tool like MassTer that can help you efficiently run measurement across multiple regions at once.
The result of this approach is a well-targeted regional campaign made solid by a statistical approach that doesn’t use up your entire budget